Learning how to run RCTs in continuous time can make for realistic treatment effects to help us make and benefit from better decisions.
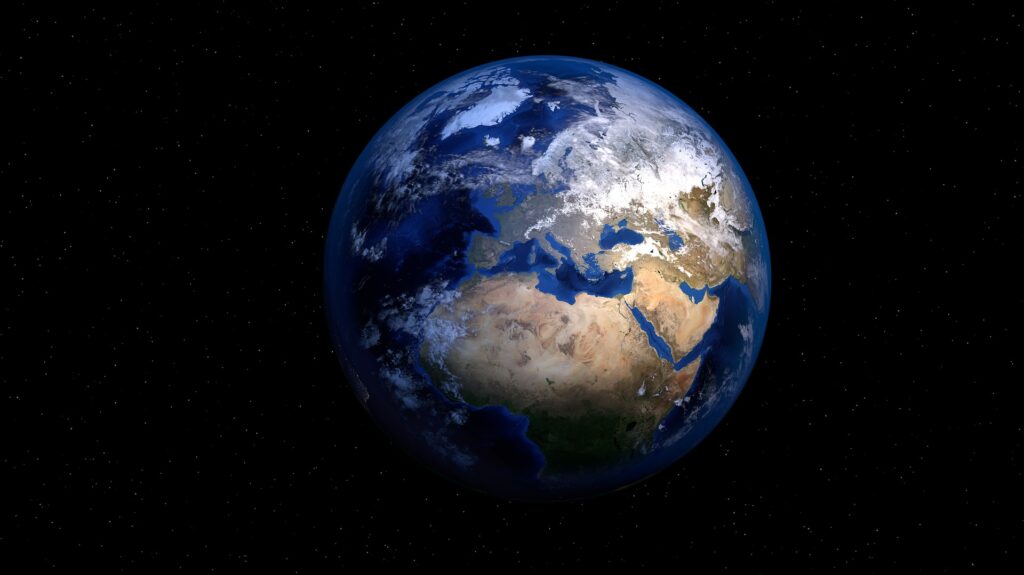
By Kweku Opoku-Agyemang.
Randomized experiments are widely used in development economics to estimate the causal effects of various interventions, such as cash transfers, microcredit, or education programs. This draws on an established history of randomized controlled trials in medicine, health and science. However, irrespective of field, most of these experiments assume that the interventions are fixed and do not change over time. This may not be realistic in many situations, where the interventions may be dynamic and depend on the context, the preferences, or the behavior of the individuals.
For example, suppose we want to evaluate the impact of a mobile app that provides personalized health advice to pregnant women. The app may send different messages to different women at different times, depending on their health status, their location, or their feedback. How can we measure the causal effect of such a time-varying intervention using a randomized experiment?
In a recent paper, Randomized Experiments in Continuous Time: A LATE for Continuous-Time Program Evaluations, from Development Economics X, I propose a general framework for estimating causal effects in continuous time using randomized experiments. I consider a setting where individuals have outcomes, treatments, and instruments that vary over time. An outcome is a variable of interest that we want to measure, such as health, income, or education. A treatment is a variable that represents the intervention that we want to evaluate, such as the app messages. An instrument is a variable that affects the treatment but not the outcome directly, such as a random assignment to receive the app or not.
The main challenge in this setting is to define what we mean by the causal effect of the treatment on the outcome in continuous time. The paper defines the potential outcomes and the causal effects in continuous time using mathematical functions that map each point in time to an outcome value. It then specifies the assumptions for identifying the local average treatment effect (LATE) using an instrument. The LATE is the average causal effect of the treatment on the outcome for those individuals who comply with the instrument. For example, the LATE of the app messages on health outcomes is the average effect for those women who use the app only because they were randomly assigned to receive it.
We show that the LATE in continuous time can be identified as a difference of ratios of conditional expectations. This means that we can estimate the LATE by comparing the expected outcomes of those who receive the treatment and those who do not, conditional on their instrument values, at each point in time. We introduce relevant identification and estimation results using both parametric and nonparametric methods. We also present extensions of the approach for heterogeneous treatment effects, multiple treatments or instruments, as well as nonparametric or semiparametric models.
Our framework allows researchers and policymakers to design and evaluate interventions that vary over time in complex settings. It also opens up new possibilities for studying dynamic phenomena in development economics, such as learning, adaptation, or behavioral change. His paper is part of a series of innovative research papers from Development Economics X, which aims to boost social impact faster and more effectively using political economy, economic history and related fields. At Development Economics X, we recognize that development economics has benefitted immensely from the experimental sciences and we attempt to give back to these fields with our innovations. Our research methodologies are also relevant for the areas of medical sciences, biotech, pharmacy and many more.
If you are interested in learning more about this topic, you can read Randomized Experiments in Continuous Time: A LATE for Continuous-Time Program Evaluations, here.
We hope you enjoyed reading our blog and learned something new about our research method. If you are interested in collaborating with us or learning more about our work, we would love to hear from you. You can contact us by email at contact@developmenteconomicsx.com and we will get back to you as soon as possible. Thank you for your time and attention. We look forward to hearing from you soon.
DEVELOPMENT ECONOMICS X. THE NEXT GENERATION
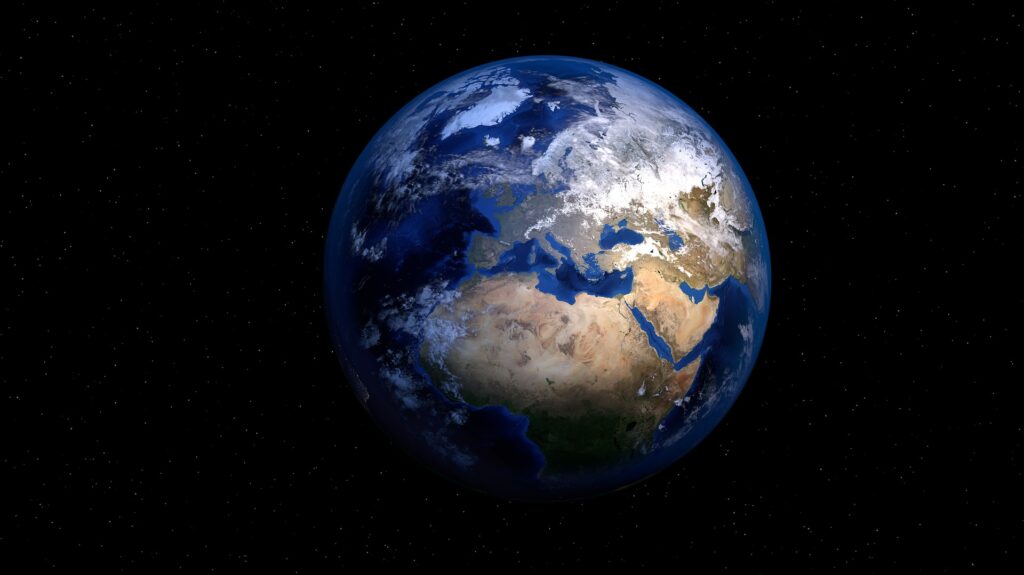